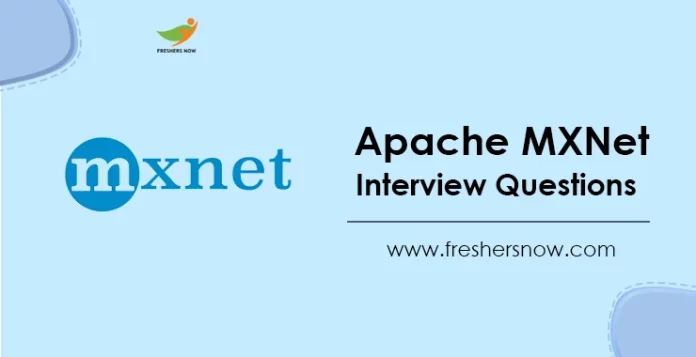
Apache MXNet Technical Interview Questions: If you’re looking to pursue a career in machine learning or artificial intelligence, then Apache MXNet is one of the popular choices for building scalable and efficient deep learning models. As an open-source deep learning framework, Apache MXNet provides a flexible and easy-to-use interface for developers and researchers. However, to land a job in this field, you need to prepare yourself for the Apache MXNet technical interview questions.
Apache MXNet Interview Questions and Answers
In this article, we have compiled the top 30 Apache MXNet interview questions and answers, including the latest Apache MXNet interview questions, Apache MXNet interview questions and answers, Apache MXNet technical interview questions, and Apache MXNet interview questions for freshers. So, let’s dive in and explore the world of Apache MXNet!
★★ Latest Technical Interview Questions ★★
Top 30 Apache MXNet Interview Questions and Answers
1. What is Apache MXNet?
Ans: Apache MXNet is an open-source deep learning framework designed to provide a scalable and efficient solution for training and deploying machine learning models. It supports multiple programming languages and has a flexible API that allows users to create custom neural network architectures.
2. What is the difference between Apache MXNet and other deep learning frameworks?
Ans: Apache MXNet is unique because it offers scalable distributed training with minimal code changes, supports multiple programming languages, and allows for easy deployment of models on various devices, including mobile devices and the cloud.
3. What programming languages does Apache MXNet support?
Ans: Apache MXNet supports a variety of programming languages, including:
- Python
- C++
- R
- Julia
- JavaScript
- and Matlab.
4. What is the role of NDArray in Apache MXNet?
Ans: NDArray is the fundamental data structure in Apache MXNet that represents tensors, vectors, and matrices used in deep learning algorithms. It allows for efficient computation of operations and can be used to represent data in various formats.
5. What are some of the most used applications of Deep Learning?
Ans: Deep Learning is used in a variety of fields. The most used ones are as follows:
- Sentiment Analysis
- Natural Language Processing
- Image Recognition
- Computer Vision
- Automatic Text Generation
- Object Detection
6. What is the role of Gluon API in Apache MXNet?
Ans: Gluon API is a high-level interface in Apache MXNet that simplifies the process of building and training neural network models. It offers an easy-to-use API for creating custom architectures and provides built-in optimization algorithms and pre-trained models.
7. What is the difference between Symbolic and Imperative programming in Apache MXNet?
Ans: Symbolic programming in Apache MXNet involves defining a symbolic graph of operations that can be optimized and compiled before execution, while imperative programming allows for dynamic execution of operations and easier debugging.
8. What is the role of a DataLoader in Apache MXNet?
Ans: A DataLoader in Apache MXNet is used to load and preprocess data for training and testing machine learning models. It can handle large datasets and provides efficient data augmentation and batching capabilities.
9. What is the role of a Loss Function in Apache MXNet?
Ans: A Loss Function in Apache MXNet measures the difference between the predicted output of a model and the actual output, and provides a signal for the model to update its parameters during training.
10. How does Apache MXNet support transfer learning?
Ans: Apache MXNet provides pre-trained models and transfer learning capabilities that allow users to reuse features learned from one model to train a new model on a different task. This can save time and resources in the training process.
11. How does Apache MXNet support model interpretation and explainability?
Ans: Apache MXNet provides built-in functionality for model interpretation and explainability, including visualization tools and algorithms for feature attribution and influence analysis.
12. What is the role of a Callback in Apache MXNet?
Ans: A Callback in Apache MXNet is a function that is called at specific points during the training process and can be used to modify the behavior of the training loop or monitor the progress of the model.
13. How does Apache MXNet handle missing data?
Ans: Apache MXNet provides built-in functions for handling missing data, including imputation and data augmentation techniques. It also supports custom data processing pipelines.
14. What is the role of a Parameter Server in Apache MXNet?
Ans: A Parameter Server in Apache MXNet is responsible for distributing and synchronizing the model parameters during distributed training. It allows for efficient communication and load balancing across multiple devices.
15. What is the difference between a Model Zoo and a Model Hub in Apache MXNet?
Ans: A Model Zoo in Apache MXNet is a repository of pre-trained models and architectures that can be used as a starting point for training new models. A Model Hub, on the other hand, is a centralized platform that hosts trained models, allowing users to easily access and deploy them for various applications.
16. How does Apache MXNet handle GPU acceleration?
Ans: Apache MXNet has native support for GPU acceleration and can leverage multiple GPUs for efficient training and inference. It also provides a GPU-aware autotuning feature that optimizes the performance of the network on specific hardware.
17. What is the role of a learning rate schedule in Apache MXNet?
Ans: A learning rate schedule in Apache MXNet is used to adjust the learning rate of the optimizer during training. It allows for a more efficient search for the optimal solution and can help avoid getting stuck in local minima.
18. How does Apache MXNet handle model deployment?
Ans: Apache MXNet provides a variety of tools and libraries for deploying trained models, including serving APIs, inference engines, and integration with popular cloud platforms like AWS and Azure.
19. What is the role of a Regularization Technique in Apache MXNet?
Ans: Regularization Techniques in Apache MXNet are used to prevent overfitting by adding constraints on the model parameters during training. This can help improve the generalization performance of the model on unseen data.
20. How does Apache MXNet handle model optimization?
Ans: Apache MXNet provides a variety of optimization algorithms, including stochastic gradient descent, Adam, and Adagrad, that can be used to efficiently train deep learning models. It also supports custom optimization strategies.
21. How does Apache MXNet handle data parallelism?
Ans: Apache MXNet uses data parallelism to distribute the workload of training across multiple devices, allowing for efficient training of large-scale deep learning models. It also provides a variety of data parallelization strategies.
22. What is the role of a ModelCheckpoint in Apache MXNet?
Ans: A ModelCheckpoint in Apache MXNet is a callback function that saves the state of the model during training at specified intervals. This can be used to resume training from a checkpoint or to select the best performing model based on validation accuracy.
23. What is the role of a CallbackMonitor in Apache MXNet?
Ans: A CallbackMonitor in Apache MXNet is a callback function that can be used to monitor various aspects of the training process, including loss, accuracy, and learning rate. It can be used to stop training early or to adjust the behavior of the training loop.
24. What is the role of a Hyperparameter Tuning in Apache MXNet?
Ans: Hyperparameter Tuning in Apache MXNet is the process of selecting the optimal values for various hyperparameters, such as learning rate, batch size, and regularization strength, to improve the performance of the model.
25. How does Apache MXNet support multi-task learning?
Ans: Apache MXNet provides built-in functionality for multi-task learning, allowing users to train a single model to perform multiple related tasks simultaneously. This can save time and resources in the training process.
26. What is the role of a CallbackMetric in Apache MXNet?
Ans: A CallbackMetric in Apache MXNet is a callback function that can be used to monitor various metrics during training, including accuracy, loss, and validation metrics. It can be used to adjust the behavior of the training loop or to stop training early.
27. What is the role of a LearningRateScheduler in Apache MXNet?
Ans: A LearningRateScheduler in Apache MXNet is a callback function that can be used to adjust the learning rate of the optimizer during training. It can be used to implement various learning rate schedules, including step decay and exponential decay.
28. How does Apache MXNet handle model serialization and deserialization?
Ans: Apache MXNet provides built-in functions for serializing and deserializing models, allowing users to save and load trained models for later use. This can be useful for deploying models in production or for sharing models with others.
29. What is the role of a Parameter Server in Apache MXNet?
Ans: A Parameter Server in Apache MXNet is a distributed system that manages the parameters of the model during training. It allows for efficient communication and synchronization between multiple workers and can improve the scalability of the training process.
30. How does Apache MXNet handle model interpretability?
Ans: Apache MXNet provides built-in tools and libraries for model interpretability, including visualization of activation maps and feature importance scores. It also supports techniques such as LIME and SHAP for explaining the predictions of black-box models.
Preparing with the help of the Top 30 Apache MXNet Interview Questions and Answers is crucial for succeeding in your technical interview and securing a job in the machine learning and artificial intelligence field. By doing so, you’ll be well-prepared to demonstrate your knowledge and skills in Apache MXNet, which can increase your chances of landing your desired job. To gain more knowledge, be sure to follow us at freshersnow.com.